Theme: Research, Knowledge exchange
Authors: Dr Matteo Ceriotti (University of Glasgow), Niven Payne (Fujitsu UK), Giulia Viavattene (University of Glasgow), Ellen Devereux (Fujitsu UK), Dr David Snelling (Fujitsu UK) and Matthew Nuckley (Fujitsu UK)
Keywords: Space, Debris Removal, Sustainability, Optimisation
Abstract: A partnership between the University of Glasgow, Fujitsu UK, Astroscale and Amazon Web Services was established in response to a UK Space Agency call on Active Debris Removal mission design. This is the process of de-orbiting space debris objects from low Earth orbit with a dedicated spacecraft. The consortium brought together different but complementary expertise and tools to develop an algorithm (using machine learning and quantum-based computing) to design multiple-debris removal missions, able to select feasible sequences of debris objects among millions of permutations, in a fraction of the time of previous methods, and of better performance in terms of time and propellant required.
Overview
Space and its services have become part of everyone’s daily life, quietly. Things like mapping, geolocation, telecommunication services and weather forecast all depend on space assets. The continuous and increasing exploration and exploitation of space heavily depends on sustainability: defunct satellites and other spacecraft and launcher parts that became part of space debris population, or “junk”, increasing the threat of collision for current and future missions. There are 34,000 objects larger than 10 cm, and 130 million smaller than 1 cm, including non-operational satellites, upper stage rocket bodies, satellite parts, etc. Most of these objects are in the low Earth orbit region (below 1000 km), which is where most satellites operate.
Design of new satellites for demise prevents the creation of further debris. Active debris removal (ADR) aims dispose of debris objects that are currently in orbit. ADR actions require a “chaser” spacecraft to grapple a “non-cooperative” target, and transfer it to an orbit low enough that it will eventually de-orbit and burn in the atmosphere in a relatively short amount of time.
The idea
Many ADR missions would be required to make a substantial contribution in diminishing the debris population. The business challenge was to investigate how we could make space debris removal missions more commercially viable. This project investigated the feasibility, viability and design of removal and disposal of multiple debris objects using a single chaser spacecraft. The mission scenario involves a spacecraft that transfers to the orbit of one or more objects, captures it (or them), and then transfers to a lower orbit for release and disposal. At low altitude, the atmospheric drag will quickly cause the object to rapidly fall and burn in the atmosphere. In the meantime, the chaser spacecraft will transfer to another object (or set of objects) and continue the mission.
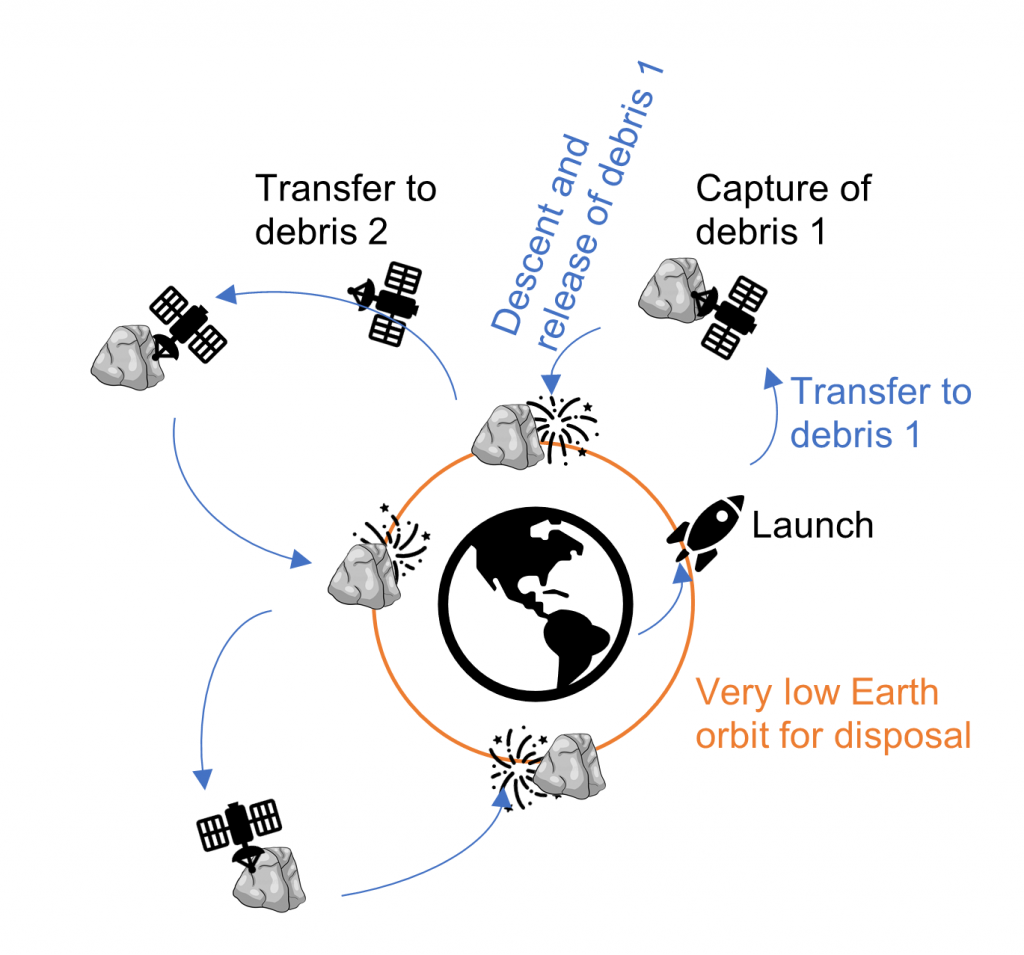
The problem
With million pieces of space junk, there are multiple trillions of permutations for ADR missions between these objects, that would need to be investigated, to efficiently remove even only a few of them. Since orbital transfers have no analytical closed-form solutions, an optimisation strategy must be used to find a solution to trajectory design problems, which is generally computationally demanding.
Our solution
The aim of this project was to make space debris removal missions more commercially viable, through a new solution that allows fast mission planning. First, an Artificial Neural Network (ANN) is trained to predict the cost of orbital transfer to and disposal of a range of debris objects quickly. Then, this information is used to plan a mission of four captures from candidate possible debris targets using Fujitsu’s quantum-inspired optimisation technology, called Digital Annealer (DA), by formulating the problem as a quadratic unconstrained binary optimisation. We used Astroscale’s mission planning data and expertise, and run the algorithms on the Amazon Web Services (AWS) Sagemaker platform. For technical details on our approach, the reader is referred to the publications below.
Outcomes
In a test-scenario, we showed that our solution produced a 25% faster mission, using 18% less propellant when compared to an expert’s attempt to plan the mission using the same assumptions; this was found 170,000 times faster than current methods based on an expert’s work.
Partnership
The project involved the partnership of four institutions, with areas of contributions described in the following diagram:
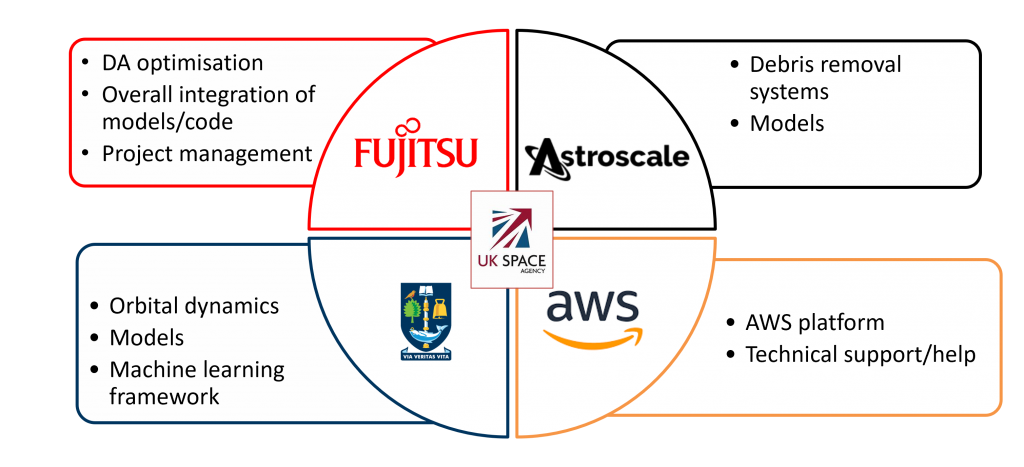
We believe the key to the success of the partnership was the different, but complementary areas of expertise, tools offered, and contribution of each partner into the project. It may be easier to rely on existing network of contacts, often with similar areas of expertise. However, this project shows that the additional effort of creating a new partnership can have great benefits, that overcome the initial difficulties.
Project set up
An initial contact between Fujitsu and UofG defined the original idea of the project, combining the existing expertise on discrete optimisation (Fujitsu) and multi-body space missions (UofG). The team was strengthened by expertise in active space debris removal (Astroscale) and cloud computing (AWS). The project proposal was funded by the United Kingdom Space Agency (UKSA), for a duration of four months, from September 2020 to January 2021.
Due to the on-going global pandemic, the project was run entirely online, with weekly meetings on Microsoft Teams. Fujitsu, as team lead, was responsible for planning and scheduling of tasks, as well as integration of code and reporting.
Lessons learned and reflections
Reactivity in preparing a project proposal was fundamental for the project: The very first contact between the partners was made at the end of July 2020, the proposal was submitted in mid-August and the project officially kicked-off in September.
Given the short timeframe, it was important to conceive a project proposal that fit the scope of the funder, but also matches with available expertise and personnel. It was also critical to frame the business challenge in the proposal.
From the point of view of the academic team, and again given the short window between notification of successful application and start of the project, these factors were crucial for the success of the project:
- Immediate availability of an internal candidate as nominated Research Assistant – there would have been no time to open a new position and recruit externally.
- An excellent researcher was particularly important, as there was no time to account for potential errors in the methods and their implementation.
- A candidate with experience aligned with the project was sought – there would have been no time to train new staff.
A PhD student in the research group was the best candidate for the project: at the cost of taking a leave-of-absence from the PhD studentship, the project constituted a unique experience with industrial collaboration, enriched their CV through a ground-breaking project, added a conference and a journal paper to their track record, and eventually opened new areas of investigation for the rest of the PhD studentship.
It would have been probably unthinkable – or at not very credible – to deliver a project with new partners remotely without any in-person meeting before the pandemic; however, this turned out to be an enabler for this project, allowing to maximise time on actual development and save on travel costs.
Further information
G. Viavattene, E. Devereux, D. Snelling, N. Payne, S. Wokes, M. Ceriotti, Design of multiple space debris removal missions using machine learning, Acta Astronautica, 193 (2022) 277-286. DOI: 10.1016/j.actaastro.2021.12.051
D. Snelling, E. Devereux, N. Payne, M. Nuckley, G. Viavattene, M. Ceriotti, S. Wokes, G. Di Mauro, H. Brettle, Innovation in planning space debris removal missions using artificial intelligence and quantum-inspired computing, 8th European Conference on Space Debris, ESA/ESOC, Darmstadt, Germany (Virtual Conference), 2021.
Any views, thoughts, and opinions expressed herein are solely that of the author(s) and do not necessarily reflect the views, opinions, policies, or position of the Engineering Professors’ Council or the Toolkit sponsors and supporters.